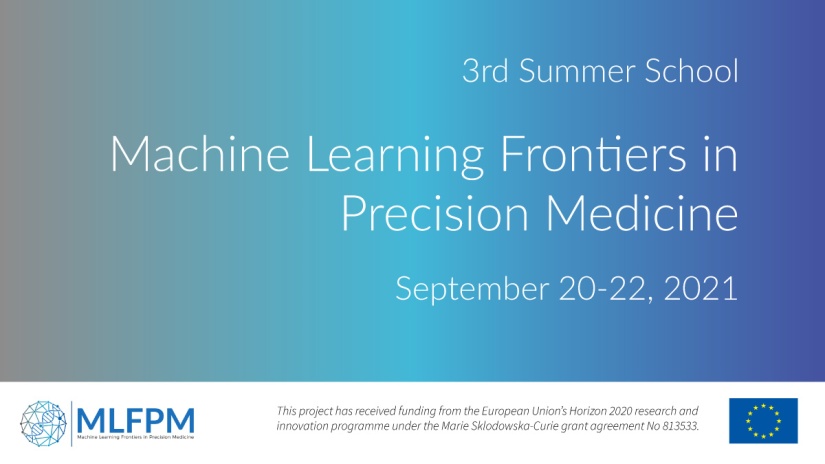
General information
Date: September 20-22, 2021
Place: ONLINE. The will be a public livestream on YouTube. No registration is needed.
Registration: No registration needed
Talks on YouTube
Recordings of all talks are available on YouTube.
Schedule
Monday, September 20
Tuesday, September 21
Wednesday, September 22
Lectures and talks
Lecture: Statistical Learning and Visualization-Defining and Looking at the Cancer Immunity State Space
Magnus Fontes (Qlucore, Institut Roche)
Monday, September 20, 08:30-10:00
Abstract: I will introduce a new basic framework for semi supervised multivariate analysis, dimension reduction and visualization of high dimensional data and exemplify how it can be used to analyze and visualize cancer immunity data.
Lecture: Machine Learning in Modern Healthcare: Challenges, Opportunities, Case Studies
Felix Agakov (Pharmatics)
Monday, September 20, 10:30-12:00
Abstract: The talk will highlight some historical and current trends in healthcare innovation, including an overview of challenges and pathways for implementing machine learning solutions in clinical practice; stakeholders’ needs; and case studies. The aim is to highlight some areas that may need to be addressed to improve the development and uptake of AI/machine learning solutions in healthcare.
Lecture: Machine learning techniques for data integration
Chloé-Agathe Azencott (Centre for Computational Biology, MINES ParisTech & Institut Curie, PSL Research University, Paris)
Monday, September 20, 13:15-14:45
Abstract: This lecture will give an overview of some techniques that can be used to learn from multi-modal data, that is to say, data sets where several complementary representations of different types (modalities) of each sample are available. Examples of different modalities include text, images or structured data, in the case of electronic health records, or single-point mutations, gene expression and methylation data, in the case of genomics.
Invited talk: Selective inference approaches for augmenting genetic association studies with multi-omics metadata
Kathryn Roeder (Department of Statistics and Data Science, Carnegie Mellon University)
Monday, September 20, 15:00-16:00
Abstract: To correct for a large number of hypothesis tests, most researchers rely on simple multiple testing corrections. Yet, new selective inference methodologies could improve power by enabling exploration of test statistics with covariates for informative weights while retaining desired statistical guarantees. We explore one such framework, adaptive p-value thresholding (AdaPT), in the context of genome-wide association studies under two types of regimes: (1) testing individual single nucleotide polymorphisms (SNPs) for schizophrenia and (2) the aggregation of SNPs into gene-based test statistics for autism spectrum disorder. We address the practical challenges of implementing AdaPT in these high-dimensional -omics settings, such as incorporating metadata with gradient boosted trees as well as adjusting for dependence induced from linkage disequilibrium (LD). To address the latter concern, we introduce an agglomerative algorithm with a linkage function determined by the LD-induced correlation structure of the gene-based test statistics. The advantages of our approaches are twofold: increased power and increased interpretability, with the latter expediting our understanding of the etiology of human diseases, disorders, and other phenotypes.
Invited talk: Omics-based risk estimates for the risk of mortality and their interpretation. The concept of biological age.
Krista Fischer (University of Tartu)
Monday, September 20, 16:30-17:30
Abstract: Mortality is the ultimate end-phenotype in epidemiology, and in some sence many others, like the incidence of chronic diseases, are surrogates for it. Therefore studies on all-cause mortality have the potential to provide unbiased estimates of the real risk-increasing factors and understanding of pathways leading to ill health, or converesely, longevity. As many population-based biobanks already have sufficiently long follow-up time for mortality, the studies on the roles of omics-based biomarkers on these pathways are providing valuable addition to the current knowledge. Although there are several statistical methods for survival analysis, and in recent years also more and more machine-learning approaches as well, translating the results to individualized risk estimates or personalized intervention strategies can often be tricky. One popular approach, is to use the concept of the biological age. A popular alternative way to illustrate biomarker effects on aging is to estimate “biological age” of the individual, where a biomarker profile may indicate that the individual is “biologically older” or “younger” than his/her actual age. In this talk, I point out that traditional ways of estimating biological age from regressing age on biomarker variables may lead to estimates that are correlated with age, but not with the actual mortality hazard. Instead, I propose an alternative definition of biological age that is directly based on survival analysis and may help to interpret individual predictions based on survival models.
Lecture: A practical guide to information extraction from medical texts
Sven Laur (University of Tartu)
Tuesday, September 21, 08:30-10:00
Abstract: Extracting certain facts from medical texts is a challenging task for many reasons. First, there are many practical hurdles like different text encodings, misspellings, unknown abbreviations. As a result, a certain set of cleaning operations must be performed before the data can be processed. Second, there are no public training sets, interesting facts are infrequent and often domain knowledge is needed to label data correctly. As a result, creating training data is far from trivial and it is almost impossible to measure recall without extensive manual labor. Third, the curse of diminishing returns often prevents us having a decent performance. It is quite easy to make initial progress, but really hard to refine your results.
In this lecture, we introduce basic methods for information extraction (rules, classifiers, transformers) and discuss how to post-process results. We also explain how to estimate the performance and how to organise the research.
Lecture: Systems analytic strategies in precision medicine
Kristel Van Steen (University of Liège)
Tuesday, September 21, 10:30-12:00
Abstract: Precision medicine and “focus on the individual” go hand in hand. Characterizations of individuals, whether healthy or not, benefit from large collections of multi-faceted data. However, the more data are collected, the higher the likelihood that redundant dependencies, but also possibly informative relationships occur. Systems analytic strategies aim to decipher these while combining data integration with interaction analysis. In this tutorial, we will address in part 1 determinants of analytic strategies that focus on interactions as crucial aspect of “systems”. In part 2 we will relate part 1 to multi-view analytic strategies that exploit (sub-)network theory. Special attention will be given to practical examples in the context of Precision Medicine, in particular the construction and potential role of individual-specific networks and microbiome systems analytics.
Invited talk: Looking into the past and future of cells: Single-cell analysis of epigenetic cell states in immunology and cancer
Christoph Bock (CeMM Research Center for Molecular Medicine of the Austrian Academy of Sciences; Institute of Artificial Intelligence, Center for Medical Statistics, Informatics, and Intelligent Systems, Medical University of Vienna)
Tuesday, September 21, 13:30-14:30
Abstract: Most diseases develop through the complex interplay of genetic and environmental influences, in-volving signaling pathways, metabolic changes, immune deregulation, and diverse cellular pheno-types. Our research is based on the hypothesis that the “epigenetic landscape” constitutes a highly informative intermediate layer of information processing that allows cells to maintain their regulatory state and cellular identity over time, while retaining the flexibility to respond swiftly to a broad range of perturbations.
In our definition, the “epigenetic landscape” is not restricted to epigenetic marks such as DNA methylation and histone modifications. Rather, it reflects the full spectrum of transcription regulation by which cells translate various inputs into sustainable changes in their cell state. Notably, the epi-genetic landscape not only reflects a cell’s current state, but also its developmental history (e.g., cell-of-origin in cancer) and its potential for future adaptation (e.g., plasticity in response to an im-munological challenge).
I will present our work within and beyond the Human Cell Atlas, dissecting epigenetic cell states in immunology and cancer; and I will present methods for causal, mechanistic analysis at scale (CROP-seq and KPNNs) and for ultra-high throughput transcriptome profiling in millions of single cells (scifi-RNA-seq).
Funding: C.B. is supported by an ERC Starting Grant (n° 679146) and an ERC Consolidator Grant (n° 101001971) of the European Union.
Invited talk: An atlas of disease-specific lifetime reproductive success
Andrea Ganna (Institute for Molecular Medicine Finland; Harvard Medical School and Massachusetts General Hospital)
Tuesday, September 21, 15:00-16:00
Abstract: Studying lifetime reproductive success, and how it is affected by diseases could provide insights into public health, social demography, and evolutionary fitness. Nordic countries such as Finland and Sweden have a long history of recording electronic health records and demographic information on a nation-wide scale, which provide unique resources for establishing a comprehensive assessment of lifetime reproductive success across a broad spectrum of diseases.
Here we use a high-throughput epidemiological approach to provide the most comprehensive picture to date on the impact of disease on lifetime reproductive success.
We obtained socio-demographic (education and income), lifetime health (ICD codes of version 8 (1969-1986), 9 (1987-1995), and 10 (1996 onward) from inpatient, outpatient, cancer, and death registries) and reproductive information (biological children including those conceived after assisted reproductive technology) for 1,723,014 Finnish and 2,521,026 Swedish alive at age 16 and never migrated abroad in the 1956-1982 birth cohort, for whom the vast majority of their reproductive cycle has been completed in 2018. The same information was also collected for their siblings, which allowed us to exploit a within-family design. In total, we included 6,963,873 individuals, for whom we obtained the status and analyzed the effects of 1,644 diseases classified in 15 categories.
To investigate the association between disease endpoints and lifetime childlessness, we performed full-sibling comparisons using conditional logistic regression models in men and women separately.
Among all diseases, mental health, physical disability, and diabetes have the largest impacts on lifetime childlessness. Sex-specific effects were observed for many diseases, with mental health generally impacting more severely in men and diabetes-related diseases impacting more severely in women. For most diseases except for infertility, the significant impacts from disease disappeared after correcting for spouseless, indicating the critical role of sexual selection. Once the individual is capable of finding a spouse and having the first child, the effects of disease on the number of subsequent children are little. Even for the same disease, the effect of disease varies with onset age. For example, most mental health diseases have stronger effects if diagnosed between age 25 and 29, which is also the peak period for finding a spouse.
In conclusion, we used nation-wide data (> 6.9 million individuals) combined with an
high-throughput unbiased approach to best control for confounding to provide the most comprehensive picture to date on the impact of disease on lifetime reproductive success.
Invited Talk: Multi-omics data integration methods for genetic diseases
Anaïs Baudot (Aix-Marseille Université, INSERM, CNRS, Marseille Medical Genetics; Barcelona Supercomputing Center)
Tuesday, September 21, 16:30-17:30
Abstract: The technological advances and accumulation of biomedical datasets are yielding unprecedented opportunities to better understand genetic diseases. However, translating massive and heterogeneous data into knowledge necessitates proper exploration and integration tools. The lecture will focus on different computational strategies for the integration of heterogeneous omics datasets. I will first describe multilayer networks that incorporate different sources of biomedical interactions, as well as associated network exploration algorithms. I will then detail joint dimensionality reduction to extract biological knowledge simultaneously from multiple omics. On the application side, I will discuss about the analysis of rare genetic diseases, which raise various challenges: many patients are undiagnosed, phenotypes can be highly heterogeneous, and only a few treatments exist.
Lecture: The various roles of machine learning in the development and use of novel digital health technologies
Florian Lipsmeier (F. Hoffmann-La Roche AG)
Wednesday, September 22, 08:30-10:00
Abstract: With the advent of digital health technologies such as ‘active’ smartphone based assessments or ‘passive’ wearable based continuous data recording during daily life, huge amounts of sensor data is being collected. Examples of sensor data are IMU (accelerometer/gyroscope/magnetometer) data streams, touch screen interactions, PPG signals, audio data, GPS, Bluetooth and many more. Irrespective of the type of data, the objective of data collection usually is maximizing the amount of information we can extract from it in the context of a given disease. Machine learning can play different crucial roles in getting us to this point. I will give an introduction to research in digital health technologies and show how we are using them in clinical studies to investigate diseases such Parkinson’s disease, Multiple Sclerosis, Schizophrenia or Autism Spectrum Disorder. In the process of unlocking this data we are leveraging Machine Learning to assess data quality, to bring context to remotely collected data from the daily life of participants, to evaluate how good dedicated hand-crafted features can predict traditional clinical assessments and to evaluate how deep-learning based features can improve upon this. I will exemplify how transferring learnings from model to model, from model to researcher and from disease to disease can help to accelerate and cross-pollinate research while keeping the amount of collected data small.
Invited Talk: Modern Hopfield Networks
Sepp Hochreiter (ELLIS Unit Linz and LIT AI Lab, Institute for Machine Learning, Johannes Kepler University Linz)
Wednesday, September 22, 10:30-11:30
Abstract: We propose a new paradigm for deep learning by equipping each layer of a deep learning architecture with modern Hopfield networks. The new paradigm is a new powerful concept comprising functionalities like pooling, memory, and attention for each layer. Associative memories date back to the 1960/70s and became popular through Hopfield Networks in 1982. Recently, we saw a renaissance of Hopfield Networks, the modern Hopfield Networks, with a tremendously increased storage capacity and an extremely fast convergence. We generalize modern Hopfield Networks with exponential storage capacity to continuous patterns. Their update rule ensures global convergence to local energy minima and they converge in one update step with exponentially low error. Surprisingly, the transformer attention mechanism is equal to the update rule of our new modern Hopfield Network with continuous states. The new modern Hopfield network can be integrated into deep learning architectures as layers to allow the storage of and access to raw input data, intermediate results, or learned prototypes. These Hopfield layers enable new ways of deep learning, beyond fully-connected, convolutional, or recurrent networks, and provide pooling, memory, association, and attention mechanisms. We demonstrate the broad applicability of the Hopfield layers across various domains. Hopfield layers improved state-of-the-art on three out of four considered multiple instance learning problems as well as on immune repertoire classification with several hundreds of thousands of instances. On the UCI benchmark collections of small classification tasks, where deep learning methods typically struggle, Hopfield layers yielded a new state-of-the-art when compared to different machine learning methods. Finally, Hopfield layers achieved state-of-the-art on two drug design datasets.
More information: GitHub, arXiv, Blog
Invited Talk: Swarm Learning: A new concept for decentralized machine learning
Joachim Schultze (German Center for Neurodegenerative Diseases (DZNE))
Wednesday, September 22, 13:30-14:30
Abstract: Fast and reliable detection of patients with severe and heterogeneous illnesses is a major goal of precision medicine. Usage of large medical data together with AI applications has to potential to revolutionize medicine. However, there is an increasing divide between what is technically possible and what is allowed, because of privacy legislation. To facilitate the integration of any medical data from any data owner worldwide without violating privacy laws, we have developed Swarm Learning—a decentralized machine-learning approach that unites edge computing, blockchain-based peer-to-peer networking and coordination while maintaining confidentiality without the need for a central coordinator, thereby going beyond federated learning. Using Swarm Learning we developed disease classifiers on distributed data in heterogeneous diseases such as COVID-19, tuberculosis, leukemia and lung pathologies. These first real-world uses cases clearly illustrated that Swarm Learning classifiers outperform those developed at individual sites. In addition, Swarm Learning completely fulfils local privacy regulations by design. We are convinced that Swarm Learning will notably accelerate the introduction of precision medicine.
Invited Talk: Medical Imaging and Analysis in the Era of Big Data
Peter Claes (KU Leuven)
Wednesday, September 22, 15:00-16:00
Abstract: Imaging genetics uses anatomical or physiological imaging technologies as dense phenotypic assays to evaluate genomic variation. It is a new emerging computational research line, aimed to impact on applied disciplines such as clinical, archeological and forensic genetics, for the benefit of patients and the society. In the post-genomics era in combination with new imaging technologies, hardware exists for intensively collecting genotypic along with phenotypic information on the level of big data. Everyday image systems are improving in resolution and imaging modalities for deep and longitudinal phenotyping. This provides a fertile ground for the deployment of artificial intelligence, machine learning and deep learning (DL) on large-scale image data, combined with strategies from statistical, quantitative, and complex genetics. In this lecture, I will focus on an overview of different biomedical imaging acquisition systems and modalities, and I will outline the main image processing and analysis techniques and how machine learning is used today to fulfill these tasks.
Invited Talk: Machine learning-based design of proteins (and small molecules and beyond)
Jennifer Listgarten (University of California, Berkeley)
Wednesday, September 22, 16:30-17:30
Abstract: Data-driven design is making headway into a number of application areas, including protein, small-molecule, and materials engineering. The design goal is to construct an object with desired properties, such as a protein that binds to a target more tightly than previously observed. To that end, costly experimental measurements are being replaced with calls to a high-capacity regression model trained on labeled data, which can be leveraged in an in silico search for promising design candidates. The aim then is to discover designs that are better than the best design in the observed data. This goal puts machine-learning based design in a much more difficult spot than traditional applications of predictive modelling, since successful design requires, by definition, some degree of extrapolation—a pushing of the predictive models to its unknown limits, in parts of the design space that are a priori unknown. In this talk, I will anchor this overall problem in protein engineering, and discuss our emerging computational approaches to tackle it.