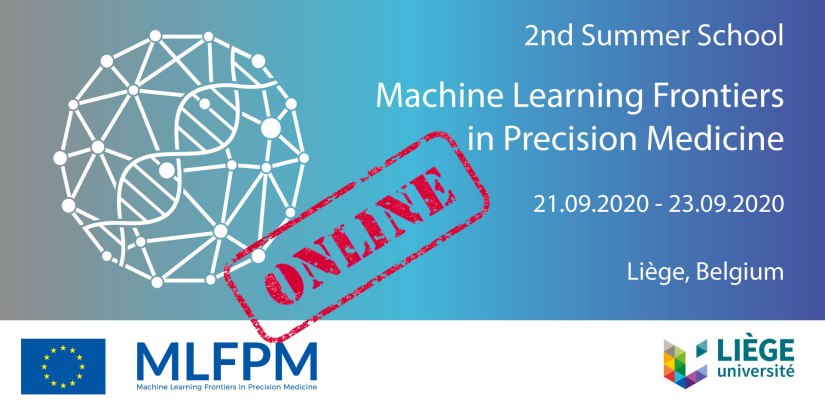
General information
Date: September 21-23, 2020
Place: ONLINE
Registration: No registration needed
Talks on YouTube
Most talks are now available on YouTube.Schedule
Time (CEST) | Monday, September 21 | Tuesday, September 22 | Wednesday, September 23 |
---|---|---|---|
09:00-10:30 | Antonio Artés-Rodríguez | Joaquin Dopazo | Caroline Uhler |
11:00-12:30 | Florence Demenais & Emmanuelle Bouzigon |
Gabriele Schweikert | Barbara Treutlein** |
13:30-14:30 | Bernhard Schölkopf | Laura Furlong | Jenna Wiens |
14:45-15:45 | Finale Doshi-Velez | Anshul Kundaje | Pearse Keane |
16:00-17:00 | Ewan Birney* | Mihaela van der Schaar | Aviv Regev |
* Ewan Birney’s talk takes place at 16:15-17:15
** Barbara Treutlein’s talk takes place at 11:00-12:00
Lectures and talks
Lecture: Probabilistic Machine Learning, Graphical and Latent Variable Models
Antonio Artés-Rodríguez (Universidad Carlos III de Madrid)
Monday, September 21, 09:00-10:30
Abstract:
This talk will provide a comprehensive overview to probabilistic machine learning and graphical models for data analysis. We will cover shallow and deep latent variable models, including Markovian ones for homogeneous and heterogenous data. Starting from the basic inference methods, we will provide an overview of the current state-of-the-art inference methods.
Lecture: Genome-Wide Association Studies (GWAS) and Post-GWAS analysis strategies
Florence Demenais (INSERM)
Emmanuelle Bouzigon (INSERM)
Monday, September 21, 11:00-12:30
Abstract:
Multifactorial diseases, such as cancers, cardiovascular diseases, asthma and allergic diseases, infectious diseases result from many genes and environmental factors and their interplays. In the last decade, genetic research on these diseases has mainly relied on Genome-Wide Association Studies (GWAS). These GWAS have been successful in identifying thousands of genetic variants (SNPs) associated with many diseases (listed in the GWAS Catalog, https://www.ebi.ac.uk/gwas/). The number of detected variants has been increasing with the sample sizes analyzed, as made possible by collaborative efforts of international consortia (allowing meta-analysis of many datasets) and access to large health resources (eg, UK Biobank among others). However, the target genes and biological effect of the disease-associated variants is still mostly unknown and these variants explain only a part of the genetic component of disease. This may be partly due to the fact that disease susceptibility results from the joint and interactive effects of many genetic factors and the effect of such factors may be missed if they are examined individually as classically done by GWAS.
A number of tools, based on functional annotations, have been proposed to identify target genes from sets of variants associated with disease in a genomic region. Strategies integrating GWAS summary statistics and various sources of biological knowledge have been developed to provide a better understanding of the biological mechanisms involved and characterization of inter-relationships among genetic factors. We will present annotation tools and data integration strategies that will be illustrated in the field of immune-related diseases and cancer.
For example, one strategy is to combine pathway analysis of GWAS outcomes and SNP-SNP interactions analysis to characterize biological pathways enriched in disease-associated genes and to discover new genes. Another strategy is to conduct network-based analysis by integrating GWAS summary statistics and protein-protein interaction networks (or any type of biological networks) to identify interconnected gene modules influencing disease. Cross-disease analysis coupled with annotation analysis also holds great promise in providing interpretation of the underlying genetic landscape of related disorders and potential drug targets.
Another use of GWAS summary data is the computation of polygenic risk scores (PRS) which can improve disease risk prediction, as will be illustrated by a few examples. GWAS summary data are also increasingly used in the context of Mendelian Randomization studies to establish a causal relationship between a disease-associated risk factor and the disease.
Thus, in the current era of high-throughput genomics which provides a growing amount of data at an unprecedented rate, integration of various types of data allows uncovering the complex mechanisms underlying multifactorial diseases and making progress towards personalized medicine.
Invited Talk: Causal machine learning: towards medical applications
Bernhard Schölkopf (Max Planck Institute for Intelligent Systems)
Monday, September 21, 13:30-14:30 CEST
Abstract:
The lecture covers basics of causality, discusses the assumption of independence causal mechanisms and the disentangled factorization of models, and will briefly visit some recent applications to Covid-19 data.
Invited Talk: Towards Using Batch Reinforcement Learning to Identify Treatment Options in Healthcare
Finale Doshi-Velez (Harvard University)
Monday, September 21, 14:45-15:45 CEST
Abstract:
In many health settings, we have available a large amounts of longitudinal, partial views of a patient (e.g. what has been coded in health records, or recorded from various monitors). How can this information be used to improve patient care? In this talk, I’ll present work that our lab has done in batch reinforcement learning, an area of reinforcement learning that assumes that the agent may access data but not explore actions. I will discuss algorithms for optimization and off-policy evaluation in the context of actual health applications, pitfalls and fundamental limitations, and how we can move forward via algorithms that engage with human experts.
This work is in collaboration with Srivatsan Srinivasan, Isaac Lage, Dafna Lifshcitz, Ofra Amir, Sonali Parbhoo, Maurizio Zazzi, Volker Roth, Xuefeng Peng, David Wihl, Yi Ding, Omer Gottesman, Liwei Lehman, Matthieu Komorowski, Aldo Faisal, David Sontag, Fredrik Johansson, Leo Celi, Aniruddh Raghu, Yao Liu, Emma Brunskill, and the CS282 2017 Course.
Invited Talk: Big data in Biology, Opportunities and Challenges
Ewan Birney (EMBL-EBI)
Monday, September 21, 16:15-17:15 CEST
Abstract:
Molecular biology is now a leading example of a data intensive science, with both pragmatic and theoretical challenges being raised by data volumes and dimensionality of the data. These changes are present in both “large scale” consortia science and small scale science, and across now a broad range of applications – from human health, through to agriculture and ecosystems. All of molecular life science is feeling this effect.
As molecular techniques – from genomics through transcriptomics and metabolomics – drop in price and turn around time there is a wealth of opportunity for clinical research and in some cases, active changes clinical practice even at this early stage. The development of this work requires inter-disciplinary teams spanning basic research, bioinformatics and clinical expertise.
This shift in modality is creating a wealth of new opportunities and has some accompanying challenges. In particular there is a continued need for a robust information infrastructure for molecular biology and clinical research. This ranges from the physical aspects of dealing with data volume through to the more statistically challenging aspects of interpreting it.
A particular opportunity is the switch from research commissioning genomic measurement to healthcare centric genomic measurement. This is occurring in a number of countries worldwide, including Australia, Denmark, Finland, France, United Kingdom and United States. The Global Alliance for Genomics and Health provides a standards setting organisation to allow for both a deepening of the technical aspects of healthcare and allowing for appropriate secondary use for research of healthcare commissioned genomics data.
I will outline the overall challenge present in this new, interdisciplinary field, and illustrate progress with specific imaging genetics results in heart from a collaboration involving my own research group.
Lecture: Mechanistic biomarkers
Joaquin Dopazo (Fundacion Progreso y Salud)
Tuesday, September 22, 09:00-10:30
Abstract:
Conventional single-gene biomarkers are easy to determine and have a demonstrated clinical utility. However, their success is purely probabilistic, often modest and frequently lack any mechanistic anchoring to the fundamental cellular processes responsible for the disease or trait of interest. This is due to the complex way in which genes interact among them to define complex phenotypes (including disease or response to drugs) and the multiple roles the genes can play, depending on the partner genes that are active. This dependence on other genes and this pleiotropy in the causal effects on the phenotypes results in imprecise determinations associated to gene-centric measurements. Conversely, the knowledge of the network of functional gene interactions that define signaling and metabolic pathways, that ultimately account for cell behavior and phenotype, and the possibility of modeling them, enables the definition of higher-level biomarkers. Such biomarkers provide a mechanistic view of biological processes in the cell and could be used to accurately predict clinically relevant endpoints. Moreover, the notion of causality associated to them enables their use for the prediction of the effect of therapeutic interventions in the system studied.
Lecture: Machine Learning for Cancer Epigenomics
Gabriele Schweikert (University of Dundee)
Tuesday, September 22, 11:00-12:30
Abstract:
In this talk we will start with a comprehensive introduction to epigenomic mechanisms, including DNA methylation, chromatin remodelling and post-translational Histone modifications. All these processes play their part in packaging and organizing the DNA molecule; they are vital for cellular differentiation and normal development and just as crucial in maintaining a specified cellular identify once cells have committed to a certain fate. Many steady-state epigenomic modifications are remarkably well correlated with transcriptional activity. However, in most cases their causal roles in the regulation of gene expression remain enigmatic.
Aberrant changes in epigenomic patterns have been observed to be hall-marks of tumorigenesis in all human cancers making them promising biomarkers for tumor development and treatment response. Additionally, genes coding for chromatin remodelers and epigenetic writers harbour some of the most frequently occurring mutations in cancer and epigenetic-targeted therapy has viable therapeutic potential for several tumor types in preclinical and clinical trials.
Here we examine the challenges that dynamic epigenomic data pose to the analysis with machine learning technology and the emerging opportunities for translational epigenomics. A particular interest is on how the interaction of epigenomic signals with other parts of the cellular machinery can be included in a mechanistic model.
Invited Talk: Enabling comorbidity analysis from real world clinical data
Laura Furlong (GRIB, Hospital del Mar Medical Research Institute (IMIM), DCEXS (UPF))
Tuesday, September 22, 13:30-14:30 CEST
Abstract:
Disease comorbidities are a major problem for public health due to their impact on quality of life, the management of patients, and healthcare cost. The increasing availability of clinical data for research (from disease cohorts, surveys and electronic health records) offers the opportunity to discover disease comorbidity as well as multimorbidity patterns from the clinical history of patients by data mining approaches. The analysis of data generated during routine medical care could then be used to improve the management of patients. In this context, approaches for the identification of disease comorbidity and multimorbidity patterns, with a special emphasis of incorporation of the temporal dimension (disease trajectory analysis), will be presented.
Invited Talk: Deep learning at base-resolution reveals motif syntax of the cis-regulatory code
Anshul Kundaje (Stanford University)
Tuesday, September 22, 14:45-15:45 CEST
Abstract:
Genes are regulated by cis-regulatory elements, which contain transcription factor (TF) binding motifs in specific arrangements. To understand the syntax of these motif arrangements and its influence on cooperative TF binding, we developed a new convolutional neural network called BPNet that models the relationship between regulatory DNA sequence and base-resolution binding profiles from ChIP-exo/nexus experiments targeting four pluripotency TFs Oct4, Sox2, Nanog, and Klf4 in mouse embryonic stem cells. BPNet is able to predict base-resolution binding profiles and footprints on sequences not used in training at unprecedented accuracy on par with replicate experiments. However, the primary appeal of neural networks for this specific application is that they are capable of learning predictive sequence representations from raw DNA sequence with minimal assumptions. Hence, interpreting these purported black box models could reveal novel insights into the cis-regulatory code. We developed a suite of model interpretation methods to learn novel motif representations, accurately map predictive motif instances in the genome and identify higher-order rules by which combinatorial motif syntax influences cooperative binding of these TFs. We discovered several novel motifs bound by these TFs supported by distinct footprints. We further found that instances of strict motif spacing are largely due to retrotransposons, but that soft motif syntax influences TF binding at protein or nucleosome range in a directional manner. Most strikingly, Nanog binding is driven by motifs with a strong preference for ~10.5 bp spacings corresponding to helical periodicity. We then validated our model’s predictions using CRISPR-induced point mutations of motif instances. The sequence representations learned by the binding models can also be seamlessly transferred to accurately predict differential chromatin accessibility after TF depletion and massively parallel reporter experiments. BPNet easily adapts to other types of profiling experiments (e.g. ChIP-seq, DNase-seq, ATAC-seq, PRO-seq), thus paving the way to decipher the complexity of the cis-regulatory code using deep learning oracle models of functional genomics data.
Invited Talk: AutoML and interpretability: powering the machine learning revolution in healthcare
Mihaela van der Schaar (University of Cambridge & UCLA)
Tuesday, September 22, 16:00-17:00 CEST
Abstract:
AutoML and interpretability are both fundamental to the successful uptake of machine learning by non-expert end users. The former will lower barriers to entry and unlock potent new capabilities that are out of reach when working with ad-hoc models, while the latter will ensure that outputs are transparent, trustworthy, and meaningful. In healthcare, AutoML and interpretability are already beginning to empower the clinical community by enabling the crafting of actionable analytics that can inform and improve decision-making by clinicians, administrators, researchers, policymakers, and beyond.
This keynote presents state-of-the-art AutoML and interpretability methods for healthcare developed in our lab and how they have been applied in various clinical settings (including cancer, cardiovascular disease, cystic fibrosis, and recently Covid-19), and then explains how these approaches form part of a broader vision for the future of machine learning in healthcare.
Lecture: Multi-Domain Data Integration: From Observations to Mechanistic Insights
Caroline Uhler (ETH Zürich & MIT)
Wednesday, September 23, 09:00-10:30 CEST
Abstract:
Massive data collection holds the promise of a better understanding of complex phenomena and ultimately, of better decisions. An exciting opportunity in this regard stems from the growing availability of perturbation / intervention data (drugs, knockouts, overexpression, etc.) in biology. In order to obtain mechanistic insights from such data, a major challenge is the integration of different data modalities (transcriptomic, proteomic, structural, etc.). I will first discuss our recent work on coupling autoencoders to integrate and translate between data of very different modalities such as sequencing and imaging. I will then present a framework for integrating observational and interventional data for causal structure discovery and characterize the causal relationships that are identifiable from such data. We end by demonstrating how these ideas can be applied for drug repurposing in the current SARS-CoV-2 crisis.
Invited Talk: Human organoid development through the lens of single-cell genomics
Barbara Treutlein (ETH Zürich)
Wednesday, September 23, 11:00-12:00 CEST
Abstract:
Recent advances in stem cell biology have made it possible to grow in vitro three-dimensional human organoids that model human brain development. We are using these organoid systems in combination with single-cell genomic methods to understand molecular mechanisms underlying fate decisions during human brain development, to explore the mechanisms underlying developmental disorders, and to identify features of organ development that are uniquely human. We deconstruct cellular composition and reconstruct differentiation trajectories over the entire course of human cerebral organoid development from pluripotency, through neuroectoderm and neuroepithelial stages, followed by divergence into neuronal fates within the dorsal and ventral forebrain, as well as midbrain and hindbrain regions. We find that the gene expression patterns in the human organoid forebrain are reproducible across iPSC lines from different individuals. We use lineage recording based on single-cell transcriptome-coupled lineage tracing, nuclei tracking from long-term light sheet microscopy, and spatial transcriptomics to understand lineage commitment and dynamics during cerebral organoid regionalization. We use single-cell CRISPR perturbation screens to identify the relevance of genes for cell fate decisions. Finally, we compare human and chimpanzee cerebral organoid development at the single-cell level to identity features that are specific to humans. Altogether, the combination of organoid technologies and single-cell technologies enable unprecedented insight into human development.
Invited Talk: From Diagnosis to Treatment – Augmenting Clinical Decision Making with Artificial Intelligence
Jenna Wiens (University of Michigan)
Wednesday, September 23, 13:30-14:30 CEST
Abstract:
Though the potential of artificial intelligence (AI) in healthcare warrants genuine enthusiasm, meaningful impact will require careful integration into clinical care. AI tools are susceptible to mistakes and rarely capable of capturing all of the nuances pertaining to a complex clinical situation. Thus, we propose approaches designed to augment, rather than replace, clinicians during clinical decision making. In this talk, I will highlight two related research directions in which we propose i) a transfer learning approach for mitigating potential shortcuts when making diagnoses and ii) a novel reinforcement learning approach for matching patients to treatments. In summary, there’s a critical need for machine learning in healthcare; however, the safe and meaningful adoption of these techniques will require collaboration between clinicians and AI.
Invited Talk: Transforming healthcare with machine learning – lessons from ophthalmology
Pearse Keane (University College London & Moorfields Eye Hospital NHS Foundation Trust)
Wednesday, September 23, 14:45-15:45 CEST
Abstract:
Ophthalmology is among the most technology-driven of the all the medical specialties, with treatments utilizing high-spec medical lasers and advanced microsurgical techniques, and diagnostics involving ultra-high resolution imaging. Ophthalmology is also at the forefront of many trailblazing research areas in healthcare, such as stem cell therapy, gene therapy, and – most recently – machine learning. In July 2016, Moorfields announced a formal collaboration with one of the world’s leading machine learning companies, DeepMind. This collaboration involves the sharing of >1,000,000 anonymised retinal scans with DeepMind to allow for the automated diagnosis of diseases such as age-related macular degeneration (AMD) and diabetic retinopathy (DR). In my presentation, I will describe the motivation – and urgent need – to apply machine learning to ophthalmology, the processes required to establish a research collaboration between the NHS and a company like DeepMind, the initial results of our research, and finally, why I believe that ophthalmology could be first branch of medicine to be fundamentally reinvented through the application of artificial intelligence.
Invited Talk: Design for Inference and the Power of Random Experiments in Biology
Aviv Regev (Broad Institute & Genentech)
Wednesday, September 23, 16:00-17:00 CEST